Should an algorithm make your healthcare decisions? With many decades of experience advocating for policies that center harm reduction and patient welfare, including as director of the International Harm Reduction Development (IHRD) Program, Daniel Wolfe believes medicine has reached an “inflection point,” as healthcare decisions are increasingly made by algorithms, not medical professionals.
Daniel’s Bellagio Reflections: “One of the great beauties of my residency was that it connected me with people who helped to enrich and expand my analytic frame. Meg Urry’s understanding of machine learning in astrophysics, and her explanation of its limitations, inspired me to test the robustness of the evidence base for the same approach in healthcare. Abril Saldaña-Tejeda and Vaishali Sinha, each separately investigating aspects of the history and deployment of reproductive technologies, urged me to pay new attention to the interplay of technological solutions with social responsibility and governance.
“They helped me recognize that it’s about much more than just picking and using a particular algorithm; it’s about the ethos that underlies it.”
Daniel Wolfe discusses the troubling outcomes that can follow when doctors defer to algorithms in making life-or-death prescription decisions.
The overdose crisis is one of the major healthcare crises in modern history, claiming more than 100,000 lives in the U.S. in 2022 alone. That’s more than the number of AIDS deaths at the height of the U.S. HIV epidemic, and more than gun violence and traffic accident deaths combined. Unfortunately, I’ve seen how a technological solution is actually creating a new, unintended crisis of its own.
In 44 states, prescribers of controlled substances are using software like NarxCare, which assigns each patient an overdose risk score based on some questionable and largely unvalidated assumptions. My work focuses on the impact of the algorithm and associated opioid control on chronic pain patients – including many who have been stable on medicine for years before having their treatment abruptly tapered or discontinued. Tens of thousands of these patients are experiencing extreme suffering as a result, with some becoming suicidal or turning to street drugs that carry increased risk of fatality.
The fundamental question at the heart of my work, and the heart of algorithmic governance in general, is whether or not these tools are fit for clinical purposes. Even though algorithms are guiding drug prescriptions and other critical medical decisions, they’re not always tested appropriately beforehand, labeled appropriately during deployment, or evaluated appropriately post-marketing. NarxCare, specifically, has never had its overdose risk score independently evaluated, while one of its other scores has only been looked at by one independent study. Despite a questionable methodology and a data set drawn from a group of mostly white patients, even that study uncovered high false positive rates for pain patients.
In fairness, the company that makes the NarxCare algorithm, Bamboo Health, is very clear that the software is only meant to guide doctors and not make decisions in and of itself. But in reality, we know that many doctors are using the algorithm’s score as a pretext for either tapering down pain medication or cutting it off altogether.
To be clear, opioid over-prescription is a real problem in the U.S., but the solution cannot be to simply cut people off. Nor should algorithmic risk scores become a substitute for physician-patient exchange in determining the course of care. An algorithm is purporting to detect what’s in a patient’s best interest without their input, and then the healthcare system makes a prescription decision based on this automated process.
I call this process “algorithmic alchemy,” where the risk score produced by an algorithm becomes more “real” and meaningful to a health system than a patient’s medical history, or anything they might say or do during a clinical consultation.
Daniel WolfeFormer director of the International Harm Reduction Development program
This moment marks an important inflection point for algorithmic decision-making, particularly in computational health – the personalization and tailoring of prevention, diagnosis, and treatment through the application of big data learnings. Over more than 20 years of working in the field of addiction, I’ve witnessed how practitioners have tended to treat everyone in largely the same way, regardless of their treatment history, comorbidities, genetics, and other individual circumstances and traits. A one-size-fits-all approach has its own terrible limits. Computation, algorithmic amalgamation and analysis of large data sets, and new approaches to both natural language processing and other kinds of predictive analytics are all incredibly powerful tools that can bring nuance to healthcare delivery, thereby transforming it.
However, I’m also aware that we’re at a moment of intense algorithmic anxiety and uncertainty. People are now talking about ChatGPT or Bard becoming the smartest doctor, teacher, or writer in any room. At the same time, people are recognizing that technologies don’t spontaneously implement themselves ethically or effectively. These innovations need to be tested in the context of existing cultures and workflows, whether in healthcare or any other setting. In other words, technologies are a tool, not a solution in and of themselves, and artificial intelligence is less important than augmented intelligence.
I don’t think we’ve achieved that balance yet, but the health sector probably has the greatest positive potential if we do. When AI is used to automate repetitive tasks, doctors can spend more time engaging with patients face-to-face. However, there’s so much work to be done to find an appropriate middle ground. We need to find a cross-disciplinary way to learn from past examples – and mistakes. Studies have found that algorithmic assessments used in the justice system for determining bail conditions often discriminate on the basis of race, criminal history, disability, and other factors. Similar biases exist in the deployment of data-driven technologies in healthcare. There needs to be a way to aggregate the lessons of this work across multiple disciplines.
I think we need to move from 20th-century regulatory structures to 21st-century ones: to data analysis, appropriate labeling and testing, and regulation. People in the machine learning or AI field often say that we need something like a Food and Drug Administration (FDA) for AI. In fact, the FDA itself needs to expand its scope and methods on algorithmic regulation. Part of my time at Bellagio involved drafting a citizen’s petition to unite scientists, legal and ethical experts, patient groups, and key analysts of algorithmic bias and governance to begin to demand at least a little more attention on this issue.
It will take some very creative thinking and engagement to realize AI’s medical promise – both with the people operating within the old system, aware of its limits, and the people who are the conceptual and creative pioneers in this space that are setting and implementing policy. But I believe it’s possible to build that bridge.
Explore more
Daniel Wolfe is the former director of the International Harm Reduction Development (IHRD) program at the Open Society Foundations, which works to support the health and human rights of people who use drugs around the world. Before this, Wolfe was a community scholar at Columbia University’s Center for History and Ethics of Public Health. Daniel attended a residency at the Bellagio Center in February 2023 with a project titled “Beyond NarxCare: From algorithmic bias to regulatory action in the U.S. overdose crisis”.
For more information about Daniel’s work, you can read about the work of the International Harm Reduction Development Program or follow Daniel on Twitter.
Related
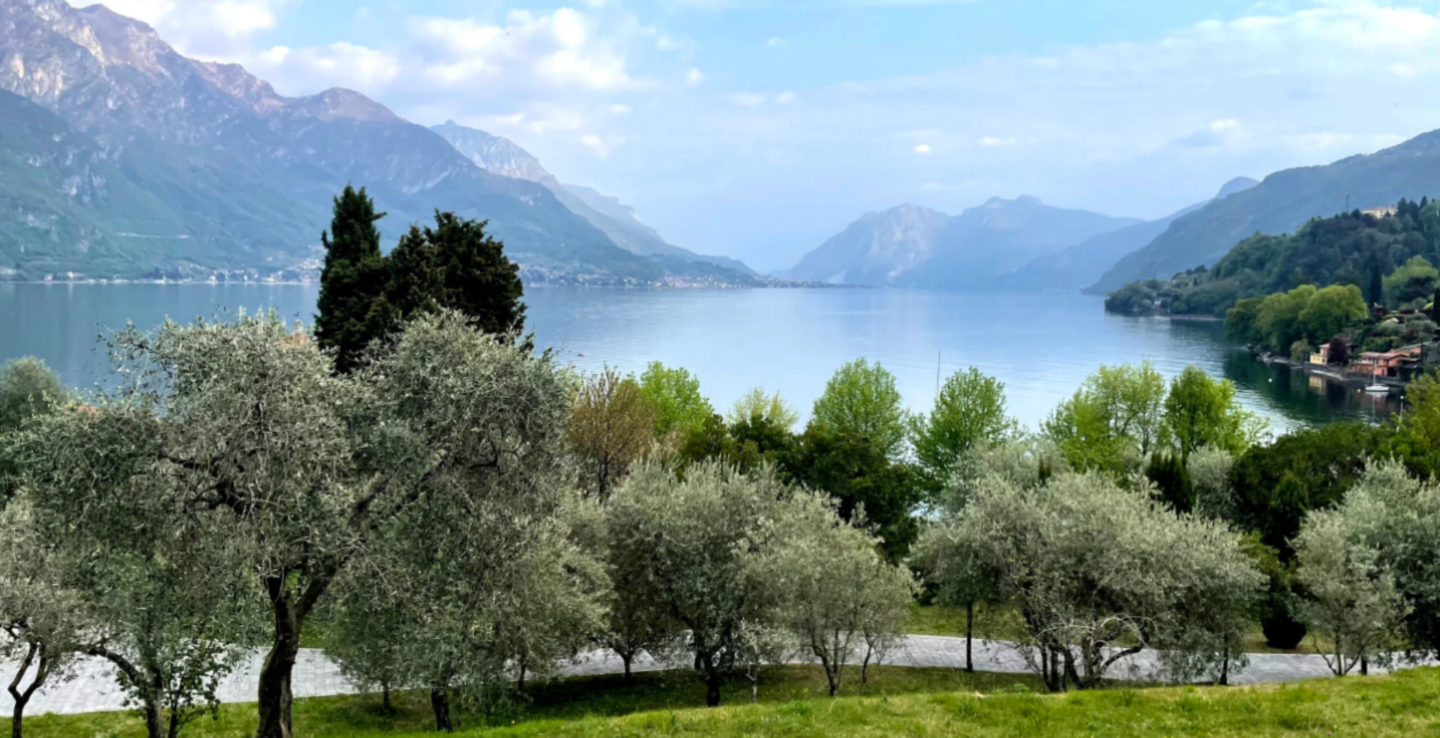
August 2023
Welcome to a special edition of the Bellagio Bulletin, where you’ll have a chance to hear from leading voices within the alumni network on one of the greatest global challenges of our time – the ethical application and governance of artificial intelligence. We hope you’ll find their points of view as illuminating as we have […]
More