We need a new profession that sits alongside machine learning engineering, one that integrates ideas from the computational, statistical, social, and behavioral sciences.
Jim GuszczaResearch affiliate at the Center for Advanced Study in the Behavioral Sciences, Stanford University
Often what’s missing is discussed in terms of “AI ethics,” but a significant stumbling block in introducing ethics to AI is not just bad intent or lack of regulation – it’s that the people who build the algorithms and the people who understand their impacts often don’t understand each other. We collectively don’t know how to bridge the gap between high-level ethical considerations and the tangible design specifications that are meaningful to engineers.
My experience as a data scientist has also taught me how difficult it can be to communicate with end-users and other impacted stakeholders, and to reflect their needs when designing algorithmic technologies. This can fail to happen even when everyone involved has good intentions. As a result, AI often reflects the narrow perspectives of its engineers. We’re seeing this with AI systems that deceive, amplify biases, or lend themselves to “off-label” uses, if not outright misuse. I especially worry about the potential of generative AI systems to accelerate the degradation of our knowledge ecosystem, analogous to how the CO2 being pumped into the atmosphere degrades our natural ecosystem. Better regulatory guardrails and better ethics are certainly necessary, but they are not sufficient. We also need smarter social norms, better choice architecture, better incentives, and better systems of human-algorithm collaboration designed into AI systems. In other words, harnessing the social and behavioral sciences is no less integral to responsible AI than harnessing machine learning and big data.
My data science experience has also taught me lessons about what I call, in machine learning, the “first-mile problem” and the “last-mile problem.” The first-mile problem is that you can’t just grab whatever data is convenient – regardless of how “big” it is. Rather, you must design the right dataset, and doing so usually has lots of ethical and domain-specific nuances. Typically it’s more of a social science challenge than a computer science challenge. The last-mile problem is that we ultimately don’t care about the algorithmic output, we care about achieving the right outcome. For example, our ultimate goal is not an accurate medical diagnostic algorithm; it is whether the patient gets better. In every real-world application of AI – from helping doctors make diagnoses, to managers making better hiring decisions, to social workers making decisions around child support – we need to start thinking through the human interaction level of this technology more systematically.
So, on the front end, social scientists and other domain experts should typically be involved in helping decide what to optimize, how to design the needed data sets, how to validate and fine-tune the models, and so on. Once we build an algorithm, we often need insights from the social and behavioral sciences to figure out how to integrate its outputs with human decisions, behaviors, and workflows. For example, it’s great that ChatGPT can predict the next words in a sentence based on a prompt, but what we ultimately want is better communication. If we’re getting a lot of misinformation and bland pastiche instead, what we have is “artificial stupidity” – not artificial intelligence.
There’s a serious need to move beyond the status quo, in which small groups of elite engineers hold a huge amount of decision-making power. We need to distribute that decision-making power more broadly – not only to domain experts and social and behavioral scientists, but also to representative stakeholders who possess crucial local knowledge of, and appreciation for, community-specific values and perspectives. So much of the rhetoric around artificial general intelligence points us away from these crucial issues. I believe that the policy community needs to step up and use its muscle to ensure that more social scientists, end-users, and stakeholders are involved in the design process of these technologies.
Ideally, we want to create processes in which computers do what computers are good at, and better enable humans to do what humans are good at. A good human-computer partnership will be one where computers compensate for natural limitations of human cognition, while humans compensate for the limitations of algorithms. This would be a huge breakthrough – but right now, all the focus is just on improving the algorithms. If we get the collaboration processes right, we’ll have something better than either the machines or the humans alone could create alone.
Explore more
Prior to CASBS, Jim was a professor at the University of Wisconsin-Madison business school, and also Deloitte Consulting’s inaugural US Chief Data Scientist. He holds a Ph.D. in Philosophy from the University of Chicago and is a Fellow of the Casualty Actuarial Society. At CASBS, he led a Rockefeller Foundation-sponsored initiative titled “Towards a Theory of AI in Practice,” which culminated in a 2022 convening at Bellagio. He returned to Bellagio for a residency in 2023 titled, “Advancing a Multidisciplinary Field of AI Practice.”
For more information about Jim’s work, visit his Deloitte profile or his CASBS profile, which also features the CASBS program “Towards a Theory of AI Practice.”
Related
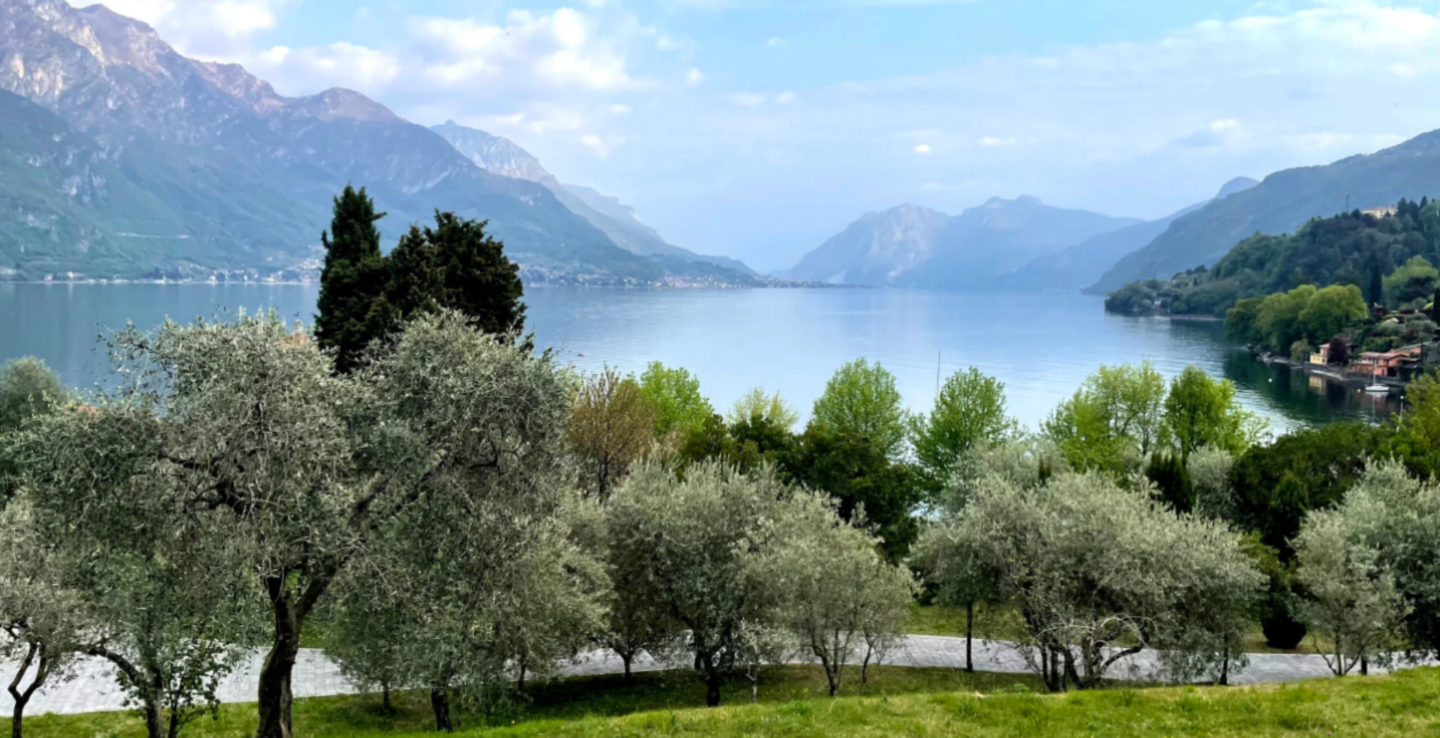
August 2023
Welcome to a special edition of the Bellagio Bulletin, where you’ll have a chance to hear from leading voices within the alumni network on one of the greatest global challenges of our time – the ethical application and governance of artificial intelligence. We hope you’ll find their points of view as illuminating as we have […]
More